‧
9 min read
The 6 most common types of bias when working with data
The Metabase Team
‧ 9 min read
Share this article
You’re trying to make a good decision, and decide to take a look at your data to help you make your call. You’ve got everything you need to feel confident, and move forward feeling invincible—after all, your data backs you up. But then, things don’t turn out the way you expect. Suddenly you’re scrambling, and trying to figure out what went wrong.
If that sounds familiar, you might be dealing with cognitive biases which are influencing and distorting how you perceive the world around you. These biases are universal —inherently a part of how everyone processes information—but they take on additional complexity when working with and learning from data.
Wait, I thought data was supposed to help me be objective?
It’s true, working with data helps you to make better decisions, anchored in reality rather than opinion. But just because you’re working with data, doesn’t mean that your biases can’t distort how you’re seeing the world.
Cognitive biases are systematic errors in thinking, usually inherited by cultural and personal experiences, that lead to distortions of perceptions when making decisions. And while data might seem objective, data is collected and analyzed by humans, and thus can be biased.
And these effects only grow when people are used to training machine learning models. The models inherit the bias of the people building them, and produce unexpected and harmful outcomes like these:
- Amazon’s recruiting machine learning system was biased against women
- Self-driving Uber car that hit and killed woman did not recognize that pedestrians jaywalk
- Racial Discrimination in Face Recognition Technology
So what can I do about data bias?
The first step to overcome bias in your decision-making is to familiarize yourself with the most common types of data bias. To get you started, we’ve collected the six most common types of data bias, along with some recommended mitigation strategies.
1. Confirmation bias
You’ve probably encountered this underlying bias every day of your life. We all love being right, so our brains are constantly on the hunt for evidence that supports our prior beliefs. Even if we’re trying our best to be open to alternative ideas, our minds are pushing back towards the safety and comfort of our own first thoughts. This can happen subconsciously through biases in how we search for, interpret, or recall information, or consciously, when we decide to cherry pick, by focusing on information that supports our arguments.
Confirmation bias example: the social media echo chamber
“I saw it on Facebook, it was shared by John” — sound familiar? Social media algorithms take advantage of people’s natural confirmation biases. By promoting and amplifying content that confirms what visitors already believe, social media platforms reinforce visitors’ prior beliefs, keeping them engaged with the platform. Users see what they already believe, and leave feeling more convinced that their views are supported in reality.
How to avoid confirmation bias
- Record your beliefs and assumptions before starting your analysis. This will help you proactively recognize your biases as you review your results.
- Go through all the presented data and evidence, but don’t immediately jump to conclusions. Resist the temptation to generate hypotheses or gather additional information to confirm your beliefs.
- Revisit your recorded beliefs and assumptions at the conclusion of your analysis, and evaluate if they’ve influenced your findings.
2. Selection bias
Selection biases occur when looking at samples that are not representative of the population. This can happen organically when working with small sets of data, or when the sampling methodology is not truly randomized.
Selection bias example: A/B test pricing
A startup wants to know whether reducing the price of their product would result in higher overall revenues. They decide to test their new pricing for a week, but only test with visitors from the US. When they roll-out the pricing to the rest of the world, they’re surprised to find that the broader audience behaves differently than their sample.
How to avoid selection bias
- Use randomization to ensure you have a representative sample rather than a convenient one.
- Make sure the samples are representative of the population in the variables you want to control (eg. Geos are evenly distributed)
3. Historical Bias
Historical data bias occurs when socio-cultural prejudices and beliefs are mirrored into systematic processes. This becomes particularly challenging when data from historically-biased sources are used to train machine learning models—for example, if manual systems give certain groups of people poor credit ratings, and you’re using that data to train the automatic system, the automatic system will replicate and may amplify the original system’s biases.
Historical bias example: word embeddings
In 2013, neural network models transformed the way machines understand written words. This technology allows computers to encode the semantic meaning of words, by learning from giant sets of written text, like Wikipedia, Google News, or Reddit. However, we’ve seen several examples where text sourced from existing datasets has produced models that mirror and amplify the existing biases contained in those datasets. For example, a machine learning model trained on Wikipedia produced gender-biased analogies like: man : doctor :: woman : nurse, or man : commander :: woman : school teacher. The model inherited the historical biases of society by learning from the huge corpora of text, and produced work further reinforcing those biases.
How to avoid historical bias
- Acknowledge and identify biases in historic and contemporary data sources.
- Establish and foster inclusivity frameworks for underrepresented groups.
4. Survivorship Bias
It’s easier to focus on the winners rather than the runners-up. If you think back to your favorite competition from the 2016 Olympics, it’s probably pretty tough to recall who got the silver and bronze. Survivorship bias influences us to focus on the characteristic of winners, due to a lack of visibility of other samples—confusing our ability to discern correlation and causation.
Survivorship bias example: Over-indexing on the advice of successful entrepreneurs
After hearing business stories from people like successful entrepreneurs, it’s tempting to try to follow their advice without any question. Why? They succeeded. You may even think that the key to being a successful entrepreneur is to never finish college.
It’s true that they achieved impressive results, but how many other people have followed the same path and failed? We pay much less attention to the silent number of entrepreneurs who failed, but there are far more of them. It doesn’t mean that we shouldn’t study the strategies of successful leaders, but we need to remember there are many more variables at play that determine which organizations succeed.
How to avoid survivorship bias
- Don’t overindex on what survived. Take a step back and consider the paths taken by both successful and unsuccessful participants.
- Find more data for the other side of the story.
5. Availability Bias
Availability of data has a big influence on how we view the world—but not all data is investigated and weighed equally. Have you ever found yourself wondering if crime has increased in your neighborhood because you’ve seen a broken car window? You’ve seen a vivid clue that something might be going on, but since you probably didn’t go on to investigate crime statistics, it’s likely that your perception shifted based on the immediately available information.
Availability bias example: Innovation mania
A breakthrough new technology is taking the world by storm. You’re seeing it on every billboard, news article, and hearing about it from your colleagues nonstop. When you encounter a problem that this technology could help you with, it’s the first thing on your mind, and you jump right into implementing it on your new project. After a few weeks, your project isn’t going as well as you’d hoped, and you realized that an older, more proven technology might have been a better solution. But because the breakthrough was easily available in your memory, you didn’t fully investigate, and ended up needing to rethink your work.
How to avoid availability bias
- Focus on larger trends and patterns, rather than vivid anecdotal outliers. It’s possible that the vivid memories you have, are the exception rather than the rule, and you can only be sure by investigating further.
- Look for different perspectives! News, media, but also your team, family, and friends play a huge role in shaping this natural shortcut, because they expose you more frequently to what they think is important for you to remember and pay attention to. You can counteract availability bias by exercising curiosity and proactively digging into available information, (even if it’s harder to get) to understand a given situation.
6. Outlier Bias
Averages are a great place to hide uncomfortable truths. Some data is convenient to visualize as an average, but this simple operation hides the effect of outliers and anomalies, and skews our observations.
Outlier Bias example: looking at the average of KPI
A start-up wants to be sure that their marketing site feels quick and responsive. They decide to track their average latency time to make sure that their site continues to load quickly. After a few months of roughly consistent average latency values, they start to see a decline in engagement on some of their most important pages. When they investigate further, they realize that the latency on those pages has skyrocketed. Their average latency time site-wide continued to paint a rosy image, because those pages were an outlier amongst many other otherwise well-performing pages.
How to avoid outlier bias
- When averages tell you things are looking good, it’s time to dig deeper.
- Look at the entire range of distribution
- Use median instead of average
- Find and investigate outliers
Final thoughts: Data bias
Working through our cognitive biases is an important part of working with and learning from data. Although data helps us see the world like never before—becoming aware of, and taking preventative measures against data biases is an important step to making better decisions with data.
Cheers,
The Metabase Team
You might also enjoy
All posts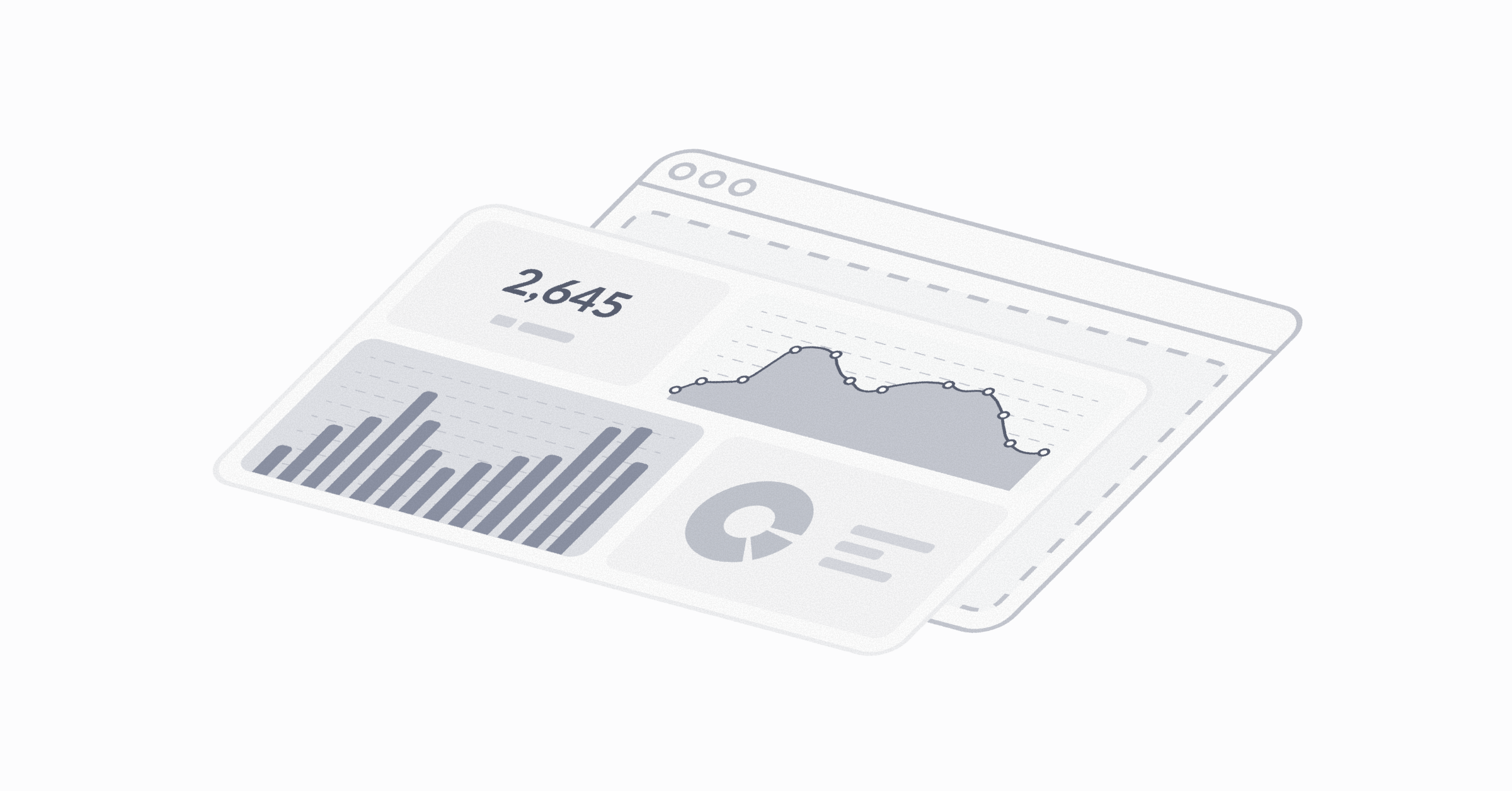
What is embedded analytics?
Embedded analytics means giving your users access to charts, metrics, and reports directly inside your product, so they can explore and act on their data without needing to leave your app or rely on someone else for answers.
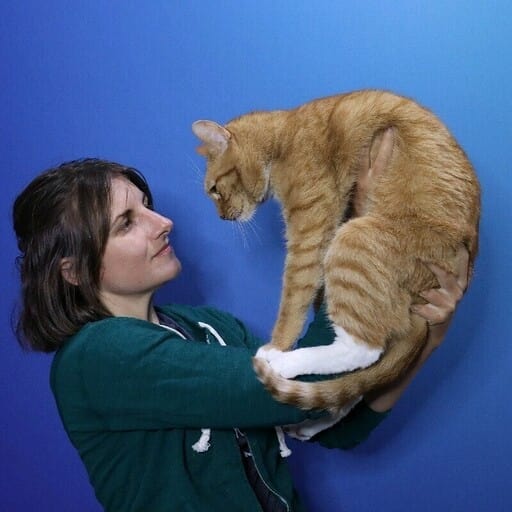
Alex Yarosh
‧
11 min read