‧
8 min read
Institutional Memory
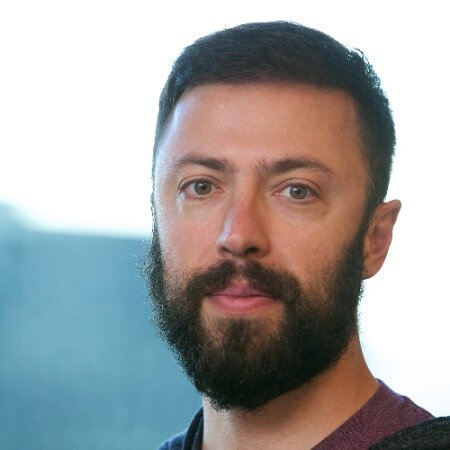
Sameer Al-Sakran
‧ 8 min read
Share this article
While a little exaggerated, anyone who’s been involved with analytics in any capacity will have some story that pretty closely matches the above story. While few people talk about it, Institutional Memory, or more typically Institutional Alzheimer’s, is one of the biggest ongoing problems in analytics at scale.
What is institutional memory?
Institutional memory is a company’s collective knowledge of what things mean, how they’ve changed, and who changed them.
Institutional Memory, or more typically Institutional Alzheimer’s, is one of the biggest ongoing problems in analytics at scale
Examples include, “how do we define an active user?,” “Do we recognize revenue on the day the contract is signed, the first day service is rendered or when their check clears?.”
Aside: In the below, we’ll refer to common numbers or sets of numbers that are computed, such as “number of active users,” as “metrics”. We’ll refer to the canonical definitions of common subsets of things as “segments”.
Why is it important?
It keeps everyone on the same page. When different people compare revenue by channel, it’s important that “revenue” and “channel” refer to the same thing. This includes both situations where two groups come up with slightly different meanings for metrics as well as cases where they used the same base reports but improvements or changes never made it to a group which is still on the old definition.
More importantly, if there are two definitions of revenue, then often one of them is just plain wrong. If you’re using an outdated or incorrect definition, then any conclusions you might draw from these revenue numbers are likely to be off.
When people compare revenue by channel, it’s important that “revenue” and “channel” refer to the same thing
It makes it easy for users who don’t understand what’s going on to pull up common numbers and apply common patterns. Most novice users aren’t going to be able to reverse engineer the definition of “active user” on their own. For them to be able to ask their own questions, it’s important that these concepts are atomic.
It also prevents chaos. One of the biggest time sucks for an analytics group (or people doing analytics work) is double checking numbers, reconciling numbers that don’t match others, and debugging reports that “seem off”. Anecdotally, this takes up at least a sizeable minority of analyst time, and in larger organizations ends up becoming a bigger time suck than actually creating dashboards or reports.
It also reduces an organization’s Bus Number. Often, there will be only one or two people in an organization that know how a particular number is computed or what a given metric really means, or the horrible horrible corners but to get a report out on time that were never revisited. Better to get this sort of information captured before they decide to take an early retirement and sail across the world with no internet connectivity.
How is it usually maintained?
Depending on how document-heavy company culture is, there are typically a variety of documents, emails and internal wiki pages describing the data model. While tedious, time consuming, error prone and generally the sort of thing that makes working in high compliance corporate environments soul crushing, this occasionally gets maintained at a satisfactory pace in a small enough organization that moves slowly enough for the documentors to catch up.
One of the biggest time sucks for an analytics group is double checking numbers
Now, the flip side of this coin is institutional memory about what common charts, reports, and dashboards mean. Here, all is hopeless chaos. Even in extremely sophisticated organizations with near unlimited resources, there’s an overwhelming number of data transformation processes written by someone no longer with the company, old reports no one has updated in months that may or may not still be used by anyone, old queries that are still being copy+pasted into new reports, and emailed spreadsheets of unclear lineage or freshness. Here, institutional memory is a literal thing: information is locked up in analysts’ and engineers’ heads.
In either case, It is very difficult and costly to keep these up to date as data models and desired analytics results change. In addition, it’s common to have these records live in a separate system from that used by end users to access data. This makes it even more likely for the reports and their documented meaning to drift apart.
How to improve institutional memory
There are two sides to improving your organization’s institutional memory.
Firstly there is just good old fashioned process and discipline. Make sure that you document definitions of metrics, what they are meant to capture, how they are computed, and their historical changes. As they change, budget time to update any central documents. Create templates or snippets for common segments (bonus points for using a tool that supports them). Often some key metrics are very difficult to measure or compute from the data available. In this case, Proxies, or numbers that behave similarly to the underlying metrics, are used. Needless to say you should definitely document how Proxies for important KPIs are calculated and their limitations.
For most of us, disciple and time are very limited. Focus on the workhorse queries and reports. Assign them an owner and make certain that it is easy to look up the person who owns the definition for any given common segment or metric. Resist the temptation to try to document everything. Create a prioritized list of common reports and concentrate on covering the top 20%. Remember that in these situations the main cost is in keeping the documents up to date over time, not writing them out the first time.
Institutional memory is a literal thing: information is locked up in analysts’ and engineers’ heads
The second main front is in tools. Some data infrastructures are more fragile than others. Using lots of different systems for the same metrics leads to confusion and maintenance load. Where possible it’s better to have a common set of tools and a repository of common reports. Standardize on a central place where people can look up information about that data model, and to whatever degree possible make that the first place people look. Spend time editing and refining this collection of information as it will save time and frustration later.
How to use Metabase to make life easier
One of the main design goals of Metabase since its early days was to help non-technical users make use of their companies databases. It is commonly acknowledged that SQL can be difficult to learn, and typical BI programs have confusing interfaces. However, much of the difficulty lies in the fact that most non-analysts don’t understand how the data model works, or how to actually ask sensible questions.
While Metabase has been designed with the goal of providing simple analytics in 5 minutes, much of the power of Metabase only comes out after the initial honeymoon winds down. Once your organization has started to see value from having a central place for dashboards, recurring reports via Pulses, and shared reports, it is time to curate your data model to allow the rest of your company to ask their own questions.
Much of the difficulty lies in the fact that most non-analysts don’t understand how the data model works
In tackling the problem of institutional memory, we’ve focused on making sure that an organization’s institutional memory lives in the place where analytics is consumed. If something is in your face every day, you’re more likely to keep it up to date. We’ve also concentrated on providing a few simple tools rather than trying to cover every case. We hope that the simple act of keeping descriptions up to date solves enough of the problem to whet user’s appetite for more. We make it easy to move from the institutional memory to the actual data or reports themselves so that it serves as a training resource.
Capturing Institutional Memory in Metabase
- Use descriptions liberally on fields that might be confusing.
- Use descriptions on tables to describe how the fields should be used, and common gotchas associated with them.
- Encapsulate common definitions of interesting entities as Segments
- For commonly graphed KPIs or otherwise interesting metrics to be reused, save them as Metrics.
- Make sure to let people know which metrics and segments they should use when you onboard them, and keep these up to date! If people know that a segment or metric is a canonical definition they’ll use it. Once it starts to slip, they’ll go back to their chaos-creating ways.
If you’re not already using Metabase, give it a whirl.