‧
8 min read
The 2015 Analytics Software Market
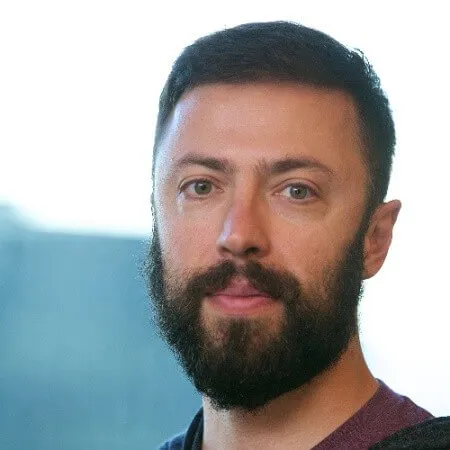
Sameer Al-Sakran
‧ 8 min read
Share this article
The 2015 Analytics Software Market
Now that the books have closed on 2015, it’s time to look back and take in some of the changes in the analytics software market. There have been several trends (open source, cloud hosting, SQL on Hadoop) that have continued to play out, as well as the emergence of AWS Redshift as a major force in data warehouses.
Additionally, a number of startups have converged around the ecological niches the emergence of Redshift has created in an otherwise stagnant market.
SQL continues its takeover of the Hadoop ecosystem
With the exception of Spark, most of the fuss in the Hadoop ecosystem is around Presto, Impala and Drill. The fight to succeed MapReduce is being fought; the major common factor in the short list of contenders is that they are based on a SQL interface. The writing has been on the wall since Hive started to edge out Pig back in 2010.
Too much of the value the Hadoop ecosystem delivers revolves around analytics and business intelligence (BI). That entire world has run on SQL for decades, and the institutional competence built around it was too much to displace. After all the NoSQL fuss with relation to Hadoop, things have gone back to where they started.
Druid seems to be picking up steam
Of the main large-scale in-memory OLAP databases, Pinot out of LinkedIn and Druid out of Metamarkets are two main players. Druid seems to be getting a fair bit of traction with Yahoo, as well as a number of tech companies on the bleeding edge increasingly using it to power real-time BI.
In October, some of the primary contributors announced Imply.io, a company that provides commercial support and will build out the ecosystem around Druid. Overall, lots of smart people are settling in on this as an in-memory database to allow interactive analysis with huge data sets.
Open source machine learning libraries galore
In November, Google open-sourced Tensor Flow , a generalized library for computing using data flow graphs. It was used heavily for machine learning and deep neural networks, specifically. It joins Theano, Torch and DMLT, which came from Microsoft in November.
While these libraries aren’t a simple plug-in to add AI to any product, they serve as foundational pieces to making state-of-the-art algorithms available to anyone with sufficient data to train their systems. As others build on the foundation, the overall sophistication of products, both analytical and otherwise, will ratchet up.
IBM throwing its weight behind Spark
IBM, in June, announced that it was putting 3,500 researchers and developers on Spark-related projects. Spark is in many ways the successor to MapReduce in the Hadoop ecosystem. It allows developers to mix and match a low-level data-processing language, a machine learning library, graph algorithms and a SQL-on-Hadoop database.
While it’s still on the bleeding edge of the adoption curve, it has massive developer support behind it. In October, IBM announced Spark as a service on Bluemix, and ported its Data Works product to Spark. What this does for Databricks, the company that has hereto had the mantle of the Apache Spark project, will be very interesting to watch.
Open-source business intelligence is having a big year
Historically, most disruption from open-source software has occurred pretty low in the software stack. With time, and the evolution of viable business models for open-source software companies, more and more end-user-facing software is being developed in the open.
Last year saw one of the two old guard open-source BI companies, Jaspersoft, acquired by Tibco for $185 million. This previous February saw the other, Pentaho, acquired by Hitachi Data Systems for more than $500 million.
Meanwhile, a number of lighterweight open-source projects emerged in 2015. AirPal and re:Dash are focused on making it quick and easy to run SQL queries on Redshift clusters (see more below), while Metabase has an easy to install tool that allows non-technical users to run queries and share dashboards and reports using data from a variety of databases.
Dedicated event analytics companies continue to chug along
While Google Analytics continues to be the default for everyone, there has continued to be a lot of activity in all-in-one analytics systems centered around collecting and analyzing user behavior on a website or mobile application.
Meanwhile, the main competitor to Google Analytics, Mixpanel (having raised $65 million to close out last year), was mostly quiet until the mid-year. In July, it followed in Heap’s footsteps and announced “Codeless Analytics.”
This is the ability to auto-instrument your mobile application by adding the SDK to your mobile application, and also get event analytics without manually instrumenting specific events like button taps. In November, it announced Predict, which lets you apply lightweight machine learning to predict whether your users will perform an action (such as a conversion).
Meanwhile, Heap has been making some noise for its ease of use in mobile and web event analytics; Amplitude raised $9 million in August; and recent entrant Interana raised a $20 million Series A in January, with a story centered around speed of analysis.
Meanwhile, the emergence of a cheap and easy way to run a data warehouse (AWS’s Redshift), has thrown a wrench in the general notion of a fully hosted, specialized event analytics offering.
The new emerging reference cloud analytics architecture
In 2015, a new standard was emerging in how growing startups (as well as companies willing to live on the medium-rare edge) were dealing with BI. There was a return to the Unified Data Warehouse concept of the 1990s. The key component that allowed this was the widespread adoption of AWS Redshift as the analytics data warehouse.
Because it is relatively easy to maintain, compared to dealing with the old school of databases (Aster, Vertica, Teradata, etc.), it quickly become the default starting point for a data warehouse for most growing tech companies.
There are two groups of startups riding this wave: those that help you get data into Redshift and those that let you analyze data once it is in Redshift.
The first group includes some companies centered entirely around loading data into Redshift (Alooma, Etleap, Textur). Additionally, Segment officially announced the ability to send data into Redshift this year. Meanwhile, RJMetrics, an e-commerce analytics provider, is now offering just the data ingestion portion of their system that lets you send data into Redshift as well.
All in all, a number of companies are hitching their wagons to Amazon, most before the QuickSight announcement came out last fall. It remains to be seen how much of a business for them remains, as AWS’s data pipelines and other ingestion services continue to improve. Given the existence of AWS’s mobile analytics SDK and ingestion pipelines, it’s a matter of will on Amazon’s part how much oxygen remains in this system in 2016.
Given the currently rudimentary capabilities shown by the preview version of QuickSight, there are a number of BI software vendors who derived a large portion of their growth in 2015 from customers heavily invested in Redshift. Looker, Mode Analytics, Periscope and Metabase have all been prominent in being used to analyze data in Redshift. Again, it remains to be seen what comes out of AWS this coming year, as well as how the landscape for these companies shift.
All in all, 2016 is looking to be a very eventful year as the implications of a funding slowdown become more apparent. While this should favor larger players, there are a number of trends very dangerous to the larger, slower incumbents.
The great tempering
While it’s always hard to tell during the thick of things, looking back, it seems pretty clear that the revenue multiple compression on the public markets side finally made its way through the snake when Fidelity publicly marked down a large number of its late-stage investments.
Of the companies relevant to analytics, Cloudera was mostly untouched while Dataminr got a valuation haircut of 35 percent. In general, capital costs for analytics startups, be they early or late-stage, got much higher. While plenty of venture firms closed new funds and have plenty of money to invest, the overall sense is that the valuations have crept downwards for given run rates or other metrics of traction.
There will be some turbulence this year in both the fundraising and, more importantly, the downstream budgets of the customer base of many analytics companies. Given how sensitive most companies’ customer lifetime values are to the C word (churn), 2016 looks to be a time to strap in and make sure you know where the airsickness bag is located.
_This post was originally published on Techcrunch _