‧
8 min read
Advice for building and scaling an effective and happy data team
The Metabase Team
‧ 8 min read
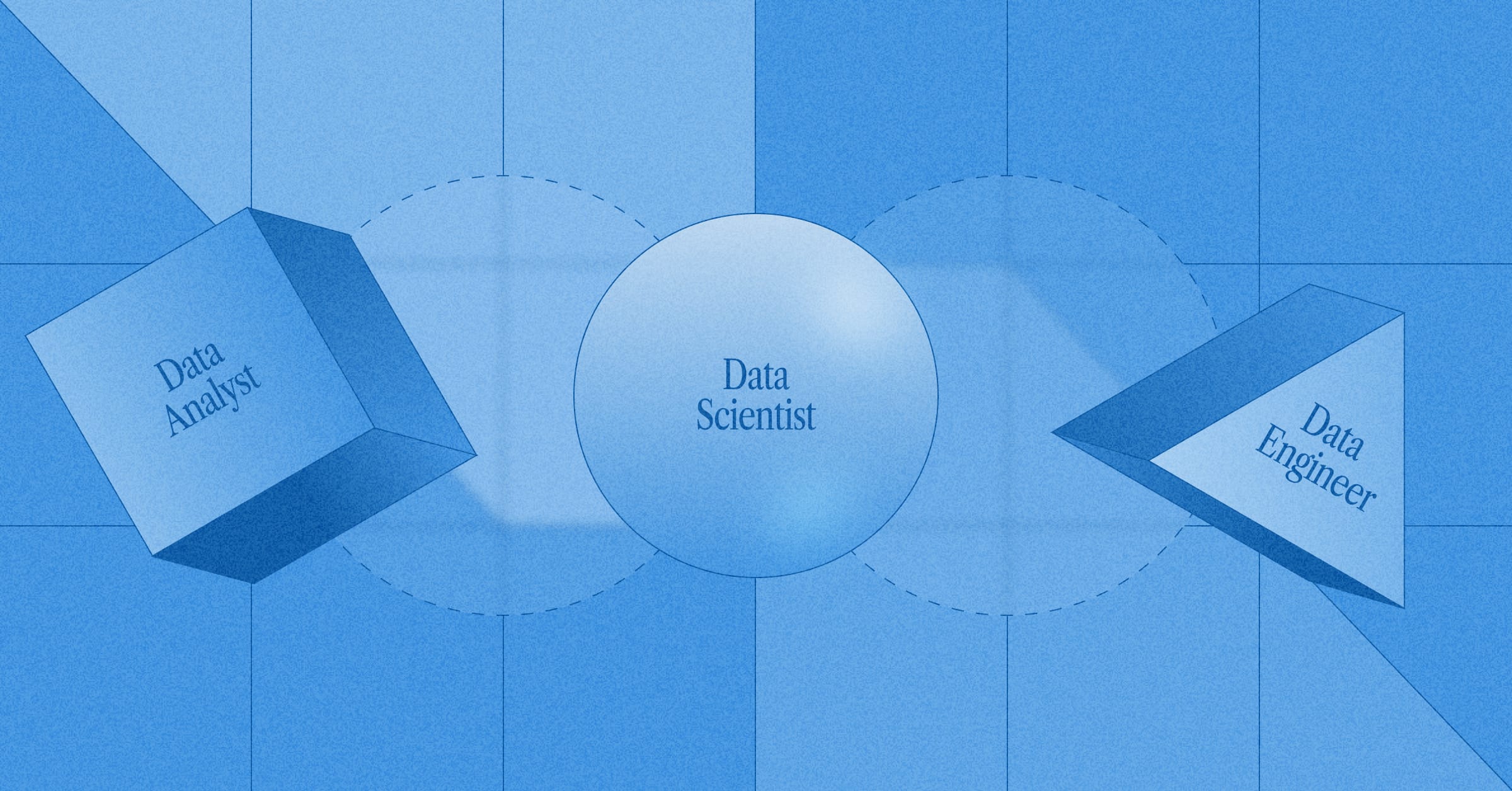
Share this article
Too many companies scope data roles beyond their capacity. It’s not uncommon to hear stories of data analysts at startups who were expected to fix pipelines and data warehousing. Or of a solo data engineer hired to contain the chaos of their company’s unwieldy modern data stack.
Is data at every company still an absolute mess?
by u/Reddit_Account_C-137 in dataengineering
Sometimes there is little understanding of the challenges data teams face, and how they do their work. But more importantly, the value they bring to a company.
If you’re a part of data team, you may know how this feels. But if you’re leading a data team, or leading a company, it can be hard to spot this issue before it spirals out of control.
We could dive into the specifics of why companies fail their data teams. But instead we’ve sourced valuable feedback, from our own data team and from data folks around the internet, to offer advice on how to confront these issues while building or scaling.
Clarify and understand business problems before collecting data
You won’t be able to solve problems if you don’t have the right data. Clarifying what questions and value you want to get from your data will help avoid common, wasteful mistakes, like investing in unnecessary tooling or infrastructure, or collecting large amounts of the wrong data.
Some data teams aren’t told what business problems to focus on, or why the company wants to collect data, and it makes it impossible for data roles to work efficiently. This can be a cause of turnover.
If you’re leading a data team or a company, explain business problems in detail to anyone in a data role. Work with the team to explain your vision for collecting and using data. Without this information, they won’t be able to help you. And it’s vital you clarify to existing employees before growing a team further. Attrition rate for all size companies has been growing since 2020.
If you’re a part of a data team, and can’t summarize the reasons why you’re collecting and working with specific data, ask management to clarify business problems and their ideas for collecting and using data. And if they don’t have the answers or time, this may be a sign that the company doesn’t have enough structure to support data culture, your role, or a data team.
Understand the strengths of each data role and delegate workload accordingly
Not setting expectations for data roles can lead to high turnover. If you’re at a smaller company or startup, you may have a limit on things like budget and team size, which make it hard to scope out roles.
However, you need to set the upper limit of each role to avoid future burnout and attrition, which can have lasting consequences for your team or company.
Before you create a new data team job description, or grow and promote your team, you should have a clear picture of gaps in coverage. Find the upper limit of each data role, and fill the gaps this way.
Roles don’t need to be scoped so definitively that an Data Analyst can never work on other things they’re interested in, but you need to set realistic boundaries for your team’s work. Or else you’ll all scramble to fill gaps in coverage, which is the quickest way to burnout.
Let’s break down some of the more common data team roles, and what their strengths are so have a clearer idea of how to scope requirements without asking for too much.
Analytics Engineers can be a good starting point for building data culture
If you’re a smaller company looking to build a culture of data, an Analytics Engineer can help clear most of the early roadblocks that data teams face when implementing new data architecture and processes.
An Analytics Engineer has the skills to bridge the gap between business requirements and team requirements. The right data engineer for this task will be able to emphasize core software skills, but with a generalist attitude and a deep empathy for business needs.
Once you have a Data Engineer on board, and pivot to the point where you need to build out custom data pipelines or optimize performance, a Data Engineer can help out.
A Data Engineer can provide specialized infrastructure while leading data governance
The Data Engineer role has changed a lot since the Hadoop era. Public cloud changed the way we look at data architecture, so a lot of Data Engineers today are expected to follow traditional SWE best practices versus data observability best practices. This can be a source of frustration.
To avoid this, know that a Data Engineer typically works on storing raw data in an appropriate data warehouse, and then providing the infrastructure and tools to other engineers, like an Analytics Engineers, to transform the data into models. They can also be responsible for optimizing storage and querying of data models by doing database and schema tuning.
Data Engineers ensure data integrity and compliance of data by adding new pipelines that bring in data from sources like APIs, and can prune obsolete pipelines that you no longer need to maintain. This is why they’re the perfect addition to your team if you’re looking for a bit of data governance.
With architecture in place and some modeled data to work with thanks to a Data Engineer, a Data Analyst can help you extract meaning from the data to start making business decisions.
Data Analysts can help make informed decisions, if you give them the room to do so
Data Analysts work with complex business problems. They’re the key to finding out truth from your data, and using the evidence to help you make decisions.
A good way to encourage analyst turnover is to hire them to start a data program from scratch, or overwhelm them with big undertakings like setting up data architecture. The Junior Data Analyst market can be heavily saturated, so it’s easy for smaller companies to make the mistake of bringing an ambitious analyst on board with the expectation of engineering work. Avoid this, and keep your analysts focused on data analysis.
The strength of a Data Analyst is their ability to see and track patterns. If you give them the space to solely focus on this, your entire company will be better equipped to understand data from different vantage points, including a customer’s point-of-view.
If you’re looking to expand into bigger data projects, like calculating future projections or ML or AI, a Data Scientist can lend a hand.
Data Scientists combine domain knowledge with their technical experience to model and validate data
Data Scientist may be the most misunderstood and debated role. Do they need the same level of in-depth engineering knowledge as a Data Engineer? Do certain companies hire Data Scientists simply because “its the thing to do”? It’s really hard to answer questions around the role of a Data Scientist as it can greatly differ per industry.
But, generally, Data Scientist roles run parallel to a Data Analyst role, and some companies use both names for the same role. A Data Scientist is typically a more technical version of data analyst, with more experience in modeling and experiments, such as machine learning.
Analysts tend to focus on understanding history (e.g., What happened to customer signups in June?), whereas Data Scientists tend to focus on building models to make predictions about the future (e.g., Given that we know X, we can create a model to predict which prospects we should reach out to, and what markets we should focus on.)
A Data Scientists strengths, like the capability to clean and preprocess data, evaluate, create, and maintain models, and validate data, can lend them to work with multiple stakeholders, from product and engineering, to finance and business leadership.
As your team continues to grow, what you do next really depends on need, but at some point if you scale into a larger company, you should consider embedding with other teams.
As you grow, data roles should embed with other teams
When a company is small, data teams tend to be more centralized or work as one team. As you grow, it will become more important to decentralize certain roles and their work.
Data and Analytics Engineers are almost always generally centralized, whereas Data Analysts and Scientists typically become more decentralized from the team as a company scales. This is because Data Analysts and Scientist need to interact with others to gain domain knowledge, and often need to available when data discrepancies and issues happen.
At this stage, the closer these roles sit to their domain area, the better their work will be. For example, if a Data Analyst’s domain area is in a specific type of product, your product, design, and engineering teams will have a lot of valuable insight on customers. Let them embed within these teams to work closely, and efficiently, together.
Keep moving toward making data available for the entire company
As you scale, self-service analytics can distribute some of the weight off of your data team. Work toward improving data literacy at the company level so everyone can learn and appreciate using data, and focus on ways to streamline ad hoc requests to avoid burnout.
Most importantly, track internal data points around data roles and processes. Data team feedback is extremely valuable. Listen to what they have to say, and work toward improving environment. It’ll have the largest impact on data culture at your company.